- Click
and then set the configurations to optimize the model’s performance, if you wish to fine-tune the configurations of your Agent.
Lookback Limit
Note: This setting is applicable only to Conversational Agents and is not applicable to Automation Agents
Define the ‘Lookback Limit’ to control how much historical chats the asset can refer to when generating responses. is crucial for tasks that benefit from contextual continuity.
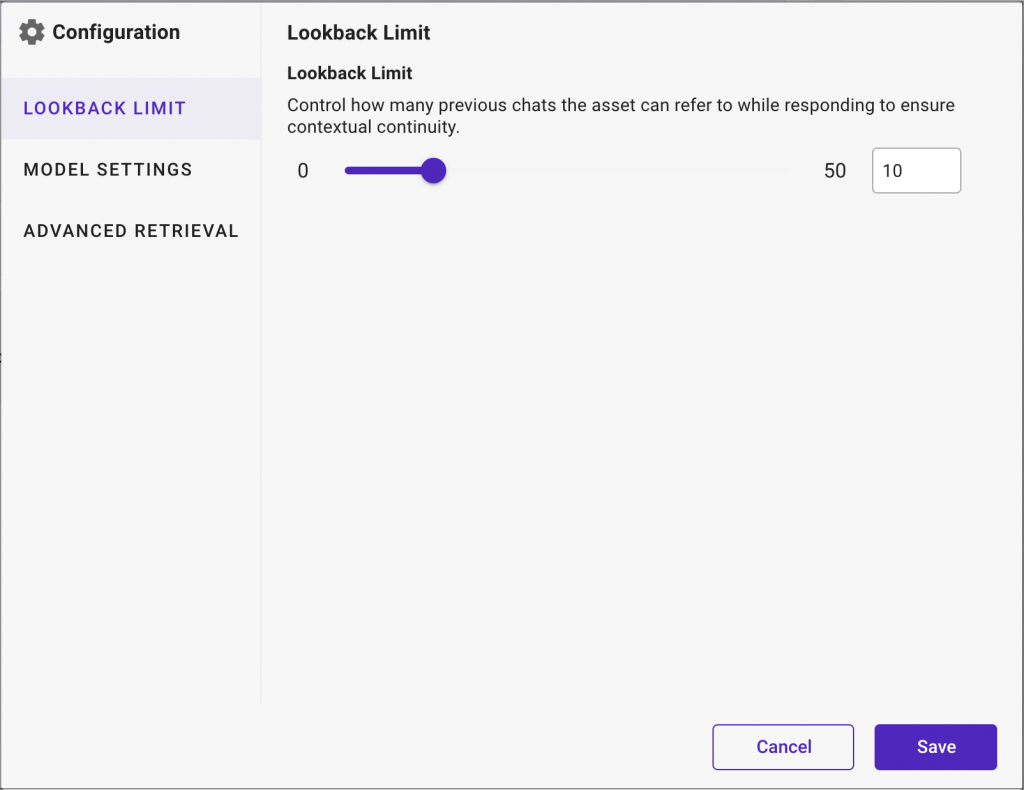
- Choose any limit from 0 to 50 and control the amount of historical chat considered.
Model Settings
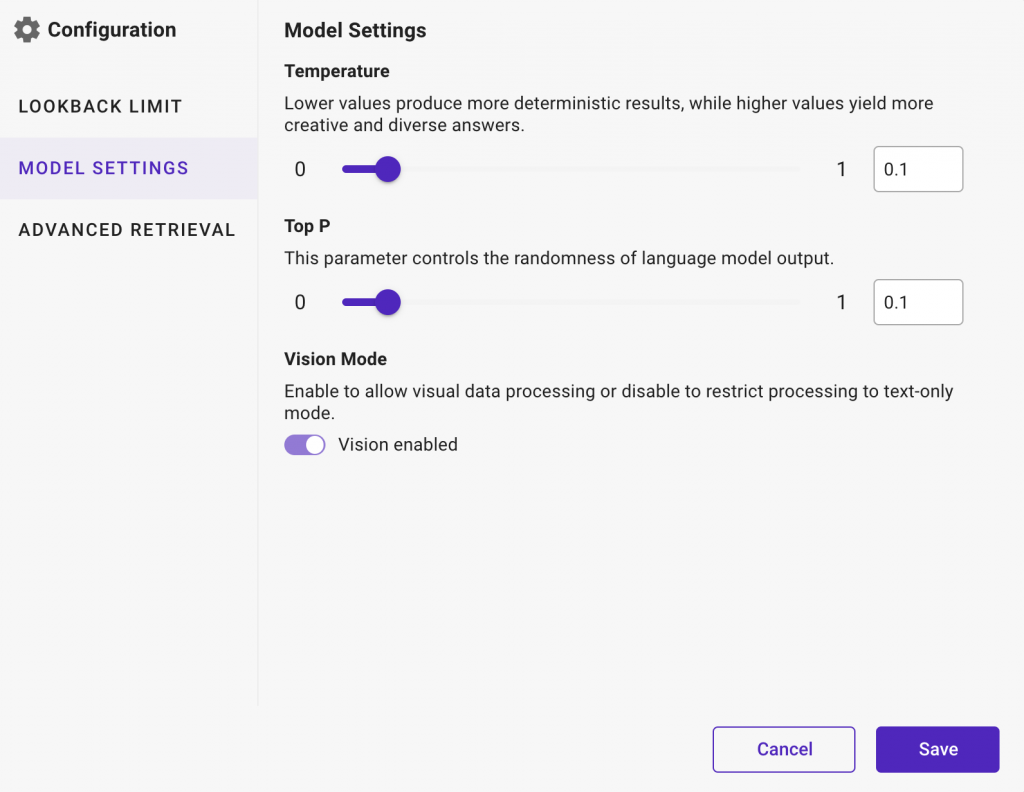
- Temperature: Choose any limit from 0 to 2 to control the randomness of the generated content.
- For example, lower values produce more deterministic results, while higher values yield more creative and diverse answers.
- For example, lower values produce more deterministic results, while higher values yield more creative and diverse answers.
- Top P: Choose any limit from 0 to 1 to set a threshold for cumulative probability during word selection, refining content by excluding less probable words.
- For example, setting top_p to 0.7 ensures words contributing to at least 70% of likely choices are considered, refining responses.
- For example, setting top_p to 0.7 ensures words contributing to at least 70% of likely choices are considered, refining responses.
- Vision (Applicable only for Bedrock Cloude3 Haiku model): Enable Vision option to decide if visual data processing is required.
Advanced Retrieval
You can configure these settings if you are using the RAG prompt template.
- Top K: Choose any limit from 1 to 20 to limit the AI model to considering only the most probable words for each token generated, aiding in controlling the generation process.
- For example, setting top_k to 10 ensures that only the top 10 most likely words are considered for each word generated.
- For example, setting top_k to 10 ensures that only the top 10 most likely words are considered for each word generated.
- Reranker: Enable the Reranker to organize initial chunks ensuring that the most relevant and accurate results are prioritized during the response generation process. This helps in refining and improving the quality of the Agent generated content by reorganizing the initial candidate answers or information chunks based on relevance criteria.
- Reranker Model: Choose any one of the following models for reranking:
- Cohere: Choose this option to develop versatile large language models (LLMs) that excel in various natural language processing (NLP) tasks such as text generation, summarization, translation, and conversational AI. These models enhance customer interactions, content creation, search capabilities, and text analysis.
- Colbert: Choose this option (Contextualized Late Interaction over BERT) to improve the relevance and accuracy of search results. It leverages advanced contextual understanding and efficient query-document matching, making it ideal for large-scale information retrieval systems.
- Cohere: Choose this option to develop versatile large language models (LLMs) that excel in various natural language processing (NLP) tasks such as text generation, summarization, translation, and conversational AI. These models enhance customer interactions, content creation, search capabilities, and text analysis.
- Top N: Choose any limit from 1 to 100 to rerank the top N most relevant and accurate chunks of information, refining output by prioritizing candidate responses or segments that best meet user needs. This setting enhances response quality by focusing on key information segments, ensuring effective and tailored content delivery.
- For example: Suppose a user asks, “Can you explain the benefits of cloud computing?” With Top N set to 3, the AI can identify and rerank the top 3 most relevant chunks of information related to cloud computing benefits. These chunks might include scalability advantages, cost-efficiency considerations, and enhanced data security features. By focusing on these key aspects, the AI delivers a well-organized and informative response that highlights the most significant benefits of cloud computing, tailored to the user’s query.
- For example: Suppose a user asks, “Can you explain the benefits of cloud computing?” With Top N set to 3, the AI can identify and rerank the top 3 most relevant chunks of information related to cloud computing benefits. These chunks might include scalability advantages, cost-efficiency considerations, and enhanced data security features. By focusing on these key aspects, the AI delivers a well-organized and informative response that highlights the most significant benefits of cloud computing, tailored to the user’s query.
- Self Query: Enable for more accurate and relevant responses using detailed metadata queries. This feature is particularly beneficial as it allows to tailor responses closely to the specific context and details provided within the query itself, leading to more accurate and useful information retrieval.
- For example: If a user asks, “How does quantum computing work?”, the Agent, utilizing Self Query, can refine its response by analyzing specific metadata within the query, such as focusing on explaining quantum computing principles and algorithms without delving into unrelated topics like classical computing, thus ensuring the response is directly tailored to the user’s query.
- For example: If a user asks, “How does quantum computing work?”, the Agent, utilizing Self Query, can refine its response by analyzing specific metadata within the query, such as focusing on explaining quantum computing principles and algorithms without delving into unrelated topics like classical computing, thus ensuring the response is directly tailored to the user’s query.
- Filtered Reference: Enables to ensure that only relevant content chunks, filtered by the LLM used in the output, are returned. It enables more effective validation of model-generated responses more effectively by focusing on the most relevant data.
This feature filters relevant sources that are actually used to generate the answer. It enables highlighting only those sources that directly contribute to the response. This feature works best with higher models like GPT 4o, GPT 4o mini & Sonnet 200k.